The world of Artificial intelligence and Machine Learning
- All Levels
- 48 hours
- 12-18 Years
-
Online Classes (Group)
INR 5000.00 Monthly
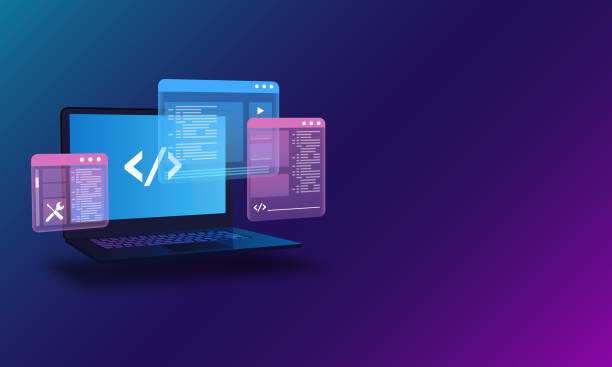
Amulya Sukrutha
India |
No votes yet
Having a strong background in the fundamentals of AI/ML is crucial for building real-world applications that automate and optimize your lifestyle. Artificial Intelligence and Machine Learning precisely describe how to train our application to learn from the data, analyze it, and gain decisive abilities.
Lesson 1: Introduction to Artificial Intelligence and Machine Learning
- What is AI? What is ML? Differences between AI, ML, DL, and Data Science
- Real-world applications
- History and evolution of AI
- Types of ML: Supervised, Unsupervised, Reinforcement Learning
- Tools setup: Python, Jupyter Notebooks, Google Colab
Lesson 2: Python for AI/ML
- Python basics recap: variables, data types, functions, control flow
- Libraries for ML: NumPy, pandas, matplotlib
- Hands-on with data loading, preprocessing, and visualization
Lesson 3: Mathematics for ML - Part 1
- Linear Algebra essentials: vectors, matrices, dot product
- Applications in ML
- Numpy-based matrix operations
Lesson 4: Mathematics for ML - Part 2
- Probability and Statistics basics
- Mean, median, variance, standard deviation
- Distributions, correlation vs. causation
Lesson 5: Supervised Learning - Regression
- Linear regression (Simple and Multiple)
- Cost function, gradient descent
- Evaluation metrics: MAE, MSE, RMSE, R² score
- Hands-on: House price prediction dataset
Lesson 6: Supervised Learning - Classification
- Logistic regression
- Decision trees
- Confusion matrix, accuracy, precision, recall, F1 score
- Hands-on: Email spam classifier
Lesson 7: Unsupervised Learning - Clustering
- K-means clustering
- Evaluation: Elbow method, silhouette score
- Hands-on: Customer segmentation
Lesson 8: Unsupervised Learning - Dimensionality Reduction
- Principal Component Analysis (PCA)
- Feature selection vs. extraction
- Hands-on: Visualizing high-dimensional data
Lesson 9: Model Evaluation and Tuning
- Train/test/validation split
- Cross-validation
- Hyperparameter tuning (Grid Search, Random Search)
- Avoiding overfitting/underfitting
Lesson 10: Introduction to Deep Learning
- What is Deep Learning? Neural Networks
- Perceptron, forward/backward propagation
- Brief intro to TensorFlow/Keras or PyTorch
Lesson 11: Capstone Project Work
- Students form groups or work individually
- Choose a dataset, define a problem, build and evaluate a model
- Instructor mentorship
Lesson 12: Project Presentations + Wrap-Up
- Students present their projects
- Peer review
- Course recap, next steps, career guidance, resources